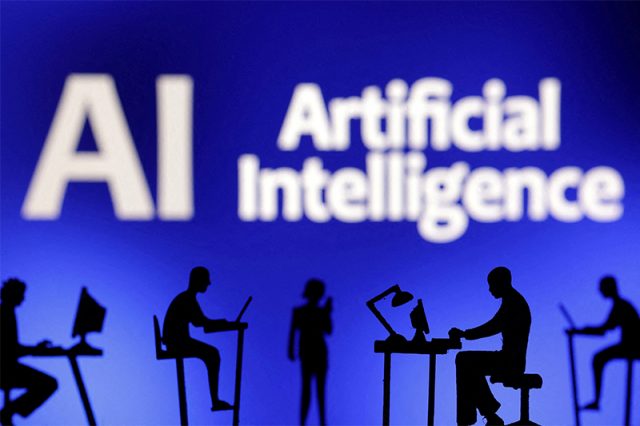
In 2024, artificial intelligence (AI) continued taking large and surprising steps forward.
People started conversing with AI “resurrections” of the dead, using AI toothbrushes and confessing to an AI-powered Jesus. Meanwhile, OpenAI, the company behind ChatGPT, was valued at US$150 billion and claimed it was on the way to developing an advanced AI system more capable than humans. Google’s AI company DeepMind made a similar claim.
These are just a handful of AI milestones over the past year. They reinforce not only how huge the technology has become, but also how it is transforming a wide range of human activities.
So what can we expect to happen in the world of AI in 2025?
Neural scaling
Neural scaling laws suggest the abilities of AI systems will increase predictably as the systems grow in size and are trained on more data. These laws have so far theorized the leap from first to second generation generative AI models such as ChatGPT.
Everyday users like us experienced this as the transition from having amusing chats with chatbots to doing useful work with AI “copilots”, such as drafting project proposals or summarising emails.
Recently, these scaling laws appear to have plateaued. Making AI models bigger is no longer making them more capable.
The latest model from OpenAI, o1, attempts to overcome the size plateau by using more computer power to “think” about trickier problems. But this is likely to increase costs for users and does not solve fundamental problems such as hallucination.
The scaling plateau is a welcome pause to the move towards building an AI system that is more capable than humans. It may allow robust regulation and global consensus to catch up.
Training data
Most current AI systems rely on huge amounts of data for training. However, training data has hit a wall as most high-quality sources have been exhausted.
Companies are conducting trials in which they train AI systems on AI-generated datasets. This is despite a severe lack of understanding of new “synthetic biases” that can compound already biased AI.
For example, in a study published earlier this year, researchers demonstrated how training with synthetic data produces models that are less accurate and disproportionately sideline underrepresented groups, despite starting with unbiased data sets.
Tech companies’ need for high-quality, authentic data strengthens the case for personal data ownership. This would give people much more control over their personal data, allowing them, for example, to sell it to tech companies to train AI models within appropriate policy frameworks.
Robotics
This year Tesla announced an AI-powered humanoid robot. Known as Optimus, this robot is able to perform a number of household chores.
In 2025, Tesla intends to deploy these robots in its internal manufacturing operations with mass production for external customers in 2026.
Amazon, the world’s second-largest private employer, has also deployed more than 750,000 robots in its warehouse operations, including its first autonomous mobile robot that can work independently around people.
Generalization – that is, the ability to learn from datasets representing specific tasks and generalize this to other tasks – has been the fundamental performance gap in robotics.
This is now addressed by AI.
For example, a company called Physical Intelligence has developed a model robot that can unload a dryer and fold clothes into a stack, despite not being explicitly trained to do so. The business case for affordable domestic robots continues to be strong, although they’re still expensive to make.
Automation
The planned Department of Government Efficiency in the United States is also likely to drive a significant AI automation agenda in its push to reduce the number of federal agencies.
This agenda is also expected to include developing a practical framework for realizing “agentic AI” in the private sector. Agentic AI refers to systems capable of performing fully independent tasks.
For example, an AI agent will be able to automate your inbox, by reading, prioritizing and responding to emails, organizing meetings and following up with action items and reminders.
Regulation
The incoming administration of newly elected US president Donald Trump plans to wind back efforts to regulate AI, starting with the repeal of outgoing president Joe Biden’s executive order on AI. This order was passed in an attempt to limit harms while promoting innovation.
Trump’s administration will also develop an open market policy where AI monopolies and other US industries are encouraged to drive an aggressive innovation agenda.
Elsewhere, however, we will see the European Union’s AI Act being enforced in 2025, starting with the ban of AI systems that pose unacceptable risks. This will be followed by the rollout of transparency obligations for generative AI models, such as OpenAI’s ChatGPT, that pose systemic risks.
Australia is following a risk-based approach to AI regulation, much like the EU. The proposal for ten mandatory guardrails for high-risk AI, released in September, could come into force in 2025.
Workplace productivity
We can expect to see workplaces continue to invest in licenses for various AI “copilot” systems, as many early trials show they may increase productivity.
But this must be accompanied with regular AI literacy and fluency training to ensure the technology is used appropriately.
In 2025, AI developers, consumers and regulators should be mindful of what Macquarie Dictionary dubbed the word of the year in 2024: enshittification.
This is the process by which online platforms and services steadily deteriorate over time. Let’s hope it doesn’t happen to AI.
Daswin de Silva, Professor of AI and Analytics, Deputy Director of the Centre for Data Analytics and Cognition, La Trobe University
This article is republished from The Conversation under a Creative Commons license. Read the original article.